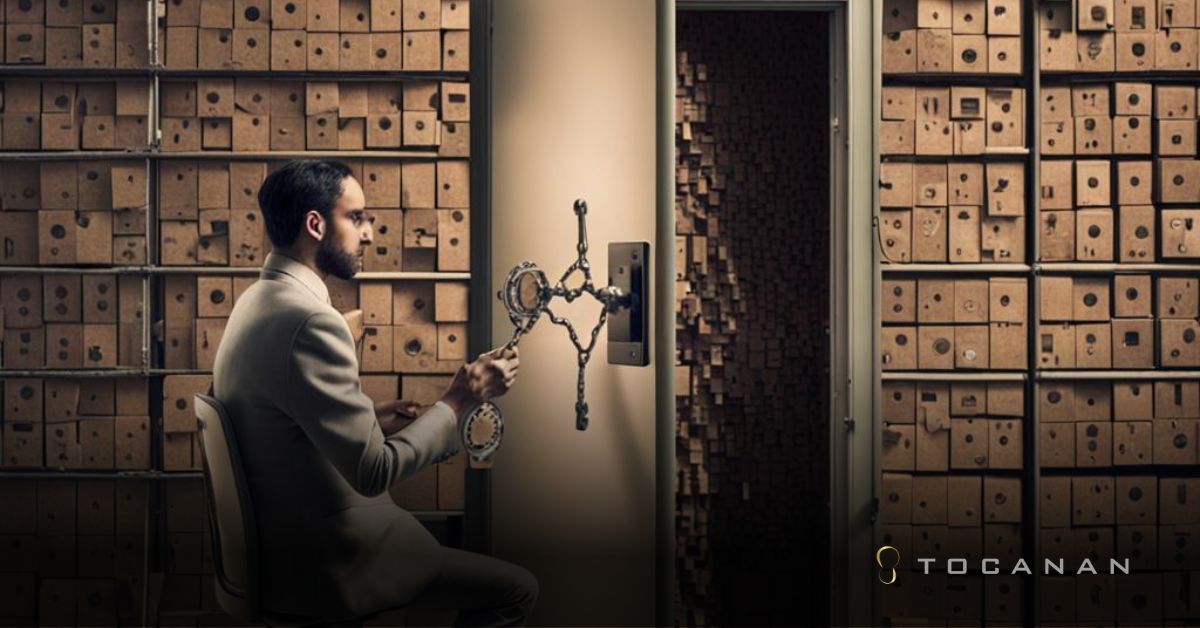
Building on our previous exploration of revolutionizing social listening with Large Language Models (LLMs), this article delves into a game-changing application of AI in marketing: effortless attribute discovery. Even seasoned brand veterans often struggle to identify the latest attributes that matter to consumers, creating significant blind spots in understanding evolving customer needs and preferences. In this piece, we’ll uncover how cutting-edge LLMs like Claude 3.5 Sonnet and GPT-4o are transforming the landscape of consumer insights, providing businesses with unparalleled access to the attributes driving customer behaviour.
I. The Importance of Attribute Understanding in Marketing
Understanding consumer sentiments involves more than just identifying whether a comment is positive or negative. It requires a granular analysis of the discussed attributes and corresponding sentiments. Attributes refer to specific product, service, or brand features that consumers mention, while sentiment analysis determines the emotional tone of these discussions. Accurate attribute identification and sentiment analysis are vital for businesses to gauge public opinion and respond appropriately.
II. The Challenge of Identifying Latest Attributes
III. LLMs: A Revolutionary Solution for Attribute Discovery
IV. Seven Steps for AI-Powered Attribute Discovery: Leveraging Coordinators and Agents
By implementing these seven steps, businesses can harness the power of AI coordinators and specialised agents to revolutionise their attribute discovery process. This advanced approach not only enhances the efficiency and accuracy of identifying product features, consumer preferences, and market trends but also provides a significant competitive edge in understanding and meeting evolving market demands. The synergy between the AI coordinator and specialised agents ensures a comprehensive, nuanced, and adaptable attribute discovery system capable of processing vast amounts of data and uncovering actionable insights.
- Intelligent AI Coordinator Implementation – Deploy an AI coordinator as the central orchestrator of the attribute discovery process. This intelligent system efficiently manages task distribution, resource allocation, and workflow optimization. The AI coordinator ensures seamless integration of various analysis components, maximizing the effectiveness of attribute identification and trend analysis across large datasets.
- Specialized AI Agents for Targeted Analysis – Implement a network of specialised AI agents, each designed for specific aspects of attribute discovery. These agents work in concert, guided by the AI coordinator, to perform tasks such as:
- a. Data preprocessing and cleaning
- b. Feature extraction
- c. Sentiment analysis
- d. Contextual interpretation
- This multi-agent approach allows for parallel processing and specialised expertise, significantly enhancing the depth and breadth of attribute discovery.
- Advanced Natural Language Processing with Transfer Learning – Leverage state-of-the-art language models within AI agents for deep textual analysis. Utilise transfer learning techniques, applying pre-trained models to specific analytical tasks. This approach enables nuanced understanding and extraction of key attributes from complex textual data, uncovering subtle trends and consumer preferences.
- Dynamic Token Management and Adaptive Chunking – Implement sophisticated chunking mechanisms within AI agents to handle extensive text volumes while adhering to model token limits. This adaptive approach ensures efficient processing of large datasets while maintaining context and coherence in the analysis, which is crucial for accurate attribute identification and trend spotting.
- Multi-dimensional Statistical Analysis – Incorporate various statistical methods within AI agents to identify prevalent themes and patterns:
- Frequency Distribution Analysis: Quantify the recurrence of attributes and themes.
- Advanced Text Vectorization: Convert textual data into numerical vectors for deeper analysis.
- Correlation Analysis: Identify relationships between different attributes and consumer behaviours. The AI coordinator aggregates these analyses for a comprehensive understanding of attribute trends.
- Unsupervised Machine Learning for Pattern Discovery – Employ clustering algorithms through specialised AI agents to group similar attributes and themes. This unsupervised approach, overseen by the AI coordinator, reveals latent structures within the text corpus, identifying patterns without predefined categories and leading to novel insights in attribute discovery.
- Scalable Architecture with Robust Error Handling – Develop an inherently scalable framework architecture that supports the AI coordinator and multiple AI agents. Incorporate comprehensive error handling and adaptive processing techniques to ensure reliable operation across diverse input scenarios. This scalable and robust design allows for easy adaptation to larger datasets and integration of new analysis types, future-proofing the attribute discovery process.
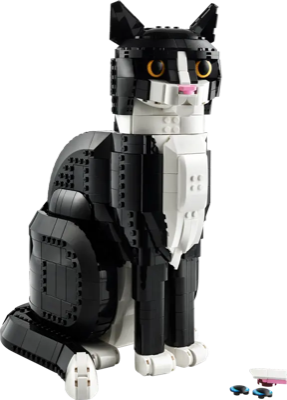
V. Case Study: Discovering User Wishes for Construction Toys
1. The Power of User-Driven Innovation in Construction Toys
In July 2024, we analysed social media to uncover player desires in the construction toy industry. This initiative collected 3,817 posts across various social media platforms, providing a dataset for our attribute discovery process.
By leveraging advanced AI-driven text analysis on these 3,817 posts, we processed this user-generated content to identify emerging trends, desires, and improvement suggestions within the community. This data-driven, user-centric approach to product development, exemplified by programs like Lego Ideas, where users submit and vote on potential new sets, demonstrates the immense value of incorporating user creativity and feedback. Our analysis has unveiled a wealth of insights, allowing for more targeted and successful innovations in the construction toy industry.
2. Key Themes and Clusters of Wishes
Our analysis revealed several key themes and clusters of wishes from construction toys enthusiasts. Below is a table summarizing the top wishes and their corresponding clusters:
Top Wishes
|
Wish Clusters
|
Customization and Creativity
|
– Enhanced Customization Options
– Compatibility with Other Brands – Increased Compatibility |
Educational and Thematic Content
|
– Educational Integration
– Diverse and Thematic Sets – Enhanced Theming and Licensing – Historical and Mythological Themes |
Quality and Durability
|
– Enhanced Durability
– Quality of Pieces – Safety |
Accessibility and Inclusivity
|
– Affordability and Accessibility
– Inclusivity and Diverse Themes |
Sustainability
|
– Environmental Sustainability
– Eco-Friendly Materials – Environmentally Friendly Materials |
Technological Integration
|
– Integration with Technology
– Enhanced Interactivity and Functionality |
User Experience Enhancements
|
– Improved Instruction Manuals
– High-Quality and Detailed Instructions |
- Customization and Creativity: Users strongly desire more flexibility in their building experiences, with enhanced customization options and better compatibility across different sets and brands.
- Educational and Thematic Content: There’s a significant interest in sets that offer educational value while also exploring diverse themes, including popular franchises, historical periods, and mythological concepts.
- Quality and Durability: Consumers consistently emphasize the importance of durable, high-quality pieces that can withstand frequent use and creative play.
- Accessibility and Inclusivity: There’s an apparent demand for more affordable sets and themes representing a broader range of cultures and experiences.
- Sustainability: Environmental concerns are increasingly important to consumers, with many expressing a desire for eco-friendly materials and sustainable practices.
- Technological Integration: Users are interested in seeing how construction toys can integrate with modern technology to enhance the building and play experience.
- User Experience Enhancements: Clear, detailed instructions remain crucial to the construction toy experience as users seek high-quality guides for complex builds.
These insights demonstrate the evolving desires of construction toy enthusiasts, highlighting areas where brands can innovate to meet consumer expectations. By addressing these clusters of wishes, companies in the construction toy industry can develop products that not only satisfy current demands but also anticipate future trends in creative play and learning.
VI. Conclusion
The integration of LLMs like Claude 3.5 Sonnet and GPT-4o into marketing practices marks a significant advancement in how businesses can understand and respond to consumer preferences. LLMs are revolutionising how brands identify and leverage key attributes by addressing the limitations of conventional attribute discovery methods and providing a deeper, more nuanced understanding of consumer discussions. As businesses embrace these advanced technologies, they will be better equipped to navigate the complexities of the modern marketplace, delivering products and experiences that truly resonate with their target audience.
Ready to unlock deep consumer insights? Request a demo now and get a free Attribute Discovery Analysis to boost your business growth.