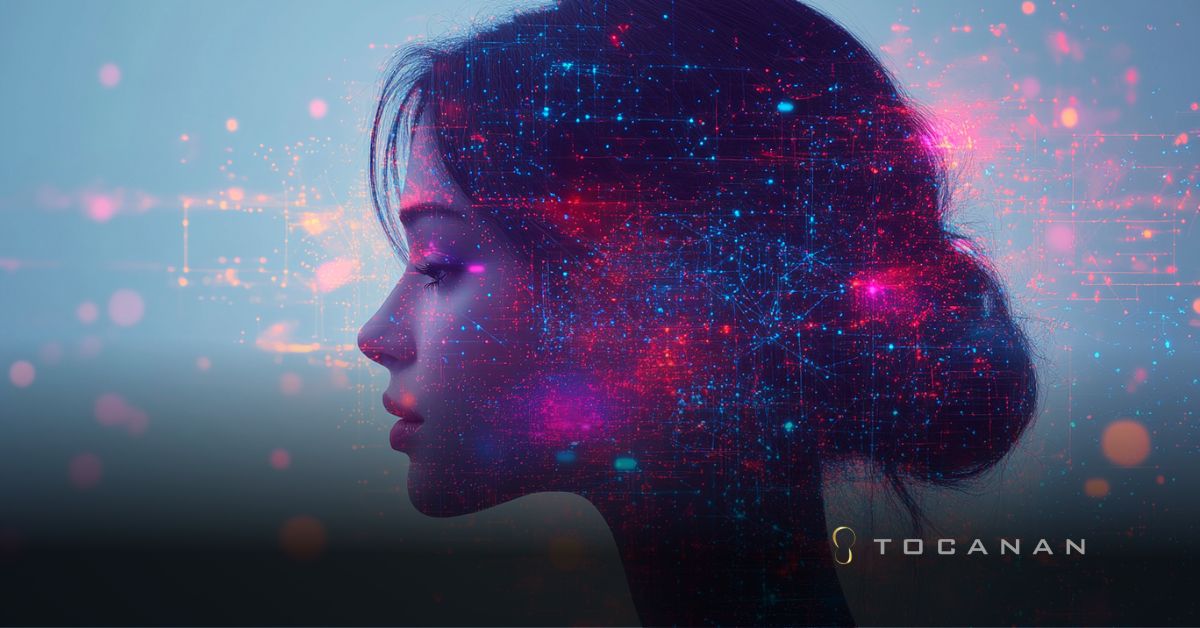
Large Language Models (LLMs) have emerged as powerful tools, not just for their ability to process and generate text, but for their increasingly sophisticated reasoning capabilities. This article explores how the reasoning power of LLMs is transforming social consumer insight businesses, enabling deeper understanding, more accurate predictions, and more nuanced analysis of consumer behavior and sentiment.
The Evolution of Reasoning in LLMs
Large Language Models, such as GPT-4o and Claude 3.5 Sonnet, have progressed beyond simple pattern recognition to demonstrate capabilities that mimic human-like reasoning in many aspects.
Key Reasoning Capabilities of LLMs:
- Contextual Understanding: LLMs can grasp complex contexts, enabling more accurate interpretation of consumer sentiment and intent.
- Logical Inference: These models can draw logical conclusions from given information, aiding in trend analysis and prediction.
- Analogical Reasoning: LLMs can draw parallels between different scenarios, helping businesses apply insights across various contexts.
- Causal Reasoning: Advanced LLMs are beginning to understand cause-and-effect relationships, crucial for strategic decision-making.
Leveraging LLM Reasoning in Social Consumer Insights
1. Advanced Sentiment Analysis with Contextual Reasoning
LLMs’ reasoning capabilities have significantly enhanced sentiment analysis, moving beyond simple positive/negative classifications.
How it works:
- LLMs analyze entire conversations, understanding context and subtext.
- They can identify sarcasm, irony, and subtle emotional cues by reasoning about the broader context.
- The models consider cultural and situational factors, leading to more nuanced sentiment classification.
Real-world example:
A global consumer electronics company uses LLM-powered sentiment analysis to understand consumer reactions to a new product launch. The LLM’s contextual reasoning allows it to differentiate between genuine enthusiasm and sarcastic praise, providing a more accurate picture of consumer reception.
2. Trend Identification and Forecasting through Logical Inference
LLMs use logical inference to identify emerging trends and predict future consumer behaviors with greater accuracy.
Capabilities:
- Analyzing social media posts, forums, and news articles to spot emerging trends.
- Using logical inference to connect disparate pieces of information and identify underlying trends.
- Predicting potential outcomes based on historical data and current trends.
Application:
An AI hardware startup utilizes LLMs to analyze discussions in tech forums and social media. The model’s reasoning capabilities allow it to infer potential future trends by connecting current discussions with historical patterns of technology adoption.
3. Personalized Customer Engagement through Analogical Reasoning
LLMs use analogical reasoning to create more personalized and relevant customer interactions, using appealing use cases, revolutionizing how businesses engage with their audience.
Features:
- Generating sophisticated product recommendations by drawing nuanced analogies between customer preferences and product attributes.
- Crafting tailored marketing messages that resonate with specific customer segments based on analogies to successful past campaigns and current market trends.
- Powering advanced conversational AI that can understand and respond to complex customer queries by drawing analogies to a vast array of past interactions.
Case study:
The innovative AI hardware startup, discussed in the last section, utilizes an LLM-powered engagement system for their pocket-sized AI assistant device. The system creates personalized marketing content by drawing analogies between the device capabilities and each potential customer’s daily routines. For busy professionals, it highlights time-saving features by analogy to their current productivity pain points. For tech enthusiasts, it draws parallels to futuristic AI concepts in popular media. This approach has not only improved customer engagement significantly but also helped position the device as a revolutionary product in the personal AI assistant market, appealing to a diverse range of consumers.
4. Content Analysis and Generation with Causal Reasoning
LLMs’ emerging causal reasoning capabilities are enhancing content analysis and generation for consumer engagement.
- Analyzing user-generated content to understand not just what consumers like or dislike, but why.
- Generating marketing content that addresses the root causes of consumer pain points or desires.
- Creating more effective A/B testing strategies by hypothesizing causal relationships between content elements and consumer responses.
Example:
A Chinese e-commerce platform review states that:
“有点黄色的脏胶,而且鞋子有瑕疵,等着穿小白鞋,失败的购物,介意大家别买,瑕疵的得物上买都要不到这个价格,差评差评” (English Translation: There’s some yellow dirty glue, and the shoes have defects. Waiting to wear white shoes. Failed purchase. Advise everyone not to buy. Even defective ones on Dewu are not this expensive. Bad review, bad review.)
The LLM’s causal reasoning analysis:
“This review reveals multiple issues with the product and their consequences. The presence of yellow glue and defects indicates quality control problems. The consumer’s expectation of ‘white shoes’ contrasts with the actual product received, leading to disappointment. The phrase ‘failed purchase’ suggests that these issues significantly impacted the overall buying experience. The comparison to 得物 (Dewu, a resale platform) prices implies that the consumer feels the product is overpriced for its quality. This chain of observations leads to the strong negative sentiment and the advice against purchasing. The causal link between product defects, pricing, and customer dissatisfaction is clear, providing valuable insights for product development and pricing strategies.”
This analysis demonstrates the LLM’s ability to identify not just individual issues, but also their interconnections and consequences, providing a deeper understanding of consumer sentiment.
5. Competitive Intelligence through Multi-Step Reasoning
LLMs’ ability to perform multi-step reasoning enhances competitive intelligence gathering and analysis.
Capabilities:
- Monitoring competitor activities across various channels and inferring strategic intentions.
- Analyzing market trends and competitor strategies to predict future moves.
- Providing insights into competitor strengths and weaknesses by connecting multiple data points.
Real-world application:
1. “平时37码的脚,这个37.5是合适的。谁能拒绝空军一号呢” (Usually wear size 37, this 37.5 fits well. Who can refuse Air Force 1?)
2. “平时37,这个36.5刚好!有一只脚鞋舌总是歪” (Usually 37, this 36.5 fits perfectly! One shoe’s tongue is always crooked)
3. “对脚比较瘦的有点偏大” (A bit large for those with slimmer feet)
- Identifies inconsistent sizing across reviews, suggesting potential sizing variability in the product.
- Notes that despite sizing issues, there’s strong brand loyalty (“Who can refuse Air Force 1?”).
- Recognizes quality control issues (crooked tongue) that coexist with fit satisfaction.
- Infers that the product may run large, especially for slim-footed consumers.
- Concludes that Nike’s Air Force 1 maintains strong market position due to brand strength, but faces challenges in consistent sizing and quality control.
- Suggests that competitors could target these weak points by focusing on consistent sizing and stricter quality control in their product development.
This multi-step reasoning demonstrates how an LLM can synthesize information from multiple sources to provide strategic insights.
6. Voice of Customer (VoC) Analysis with Abstraction and Generalization
LLMs can abstract and generalize from specific customer feedback to broader insights.
How it works:
- Analyzing customer feedback from multiple channels and abstracting common themes.
- Generalizing specific issues to broader categories of customer needs or pain points.
- Providing actionable insights by reasoning about the implications of customer feedback for different aspects of the business.
Example:
Analyzing China e-commerce platform multiple reviews:
1. “有点硬,其他没毛病” (A bit hard, no other issues)
2. “感觉有点硬” (Feels a bit hard)
3. “就是鞋有点硬,其他都还好” (The shoe is a bit hard, everything else is fine)
4. “硬,这个季节有点热了” (Hard, it’s a bit hot this season)
“These reviews collectively point to a recurring issue with the hardness of the shoes. The consistency of this feedback across multiple customers suggests it’s a significant characteristic of the product rather than isolated experiences. However, the moderate language used (‘有点‘ – a bit) and the generally positive or neutral comments about other aspects indicate that this hardness, while noticeable, isn’t severely impacting overall satisfaction.”
7. Crisis Management with Scenario Planning
LLMs’ reasoning capabilities enable more sophisticated scenario planning for crisis management.
Features:
- Real-time analysis of social media posts and comments to identify potential crises.
- Using causal and logical reasoning to predict how different response strategies might play out.
- Generating multiple scenario plans based on different potential outcomes.
Case study:
For an outdoor installation art project, organizers utilized an LLM for crisis management planning. The model analyzed various scenarios involving weather conditions, structural engineering challenges, and public safety concerns. By processing this complex data, the LLM generated various potential outcomes and suggested effective mitigation strategies, striking a balance between artistic vision, public safety, and logistical feasibility. This approach empowered organizers to make swift, informed decisions, ultimately ensuring the success and safety of the art installation in a dynamic urban environment.
Future Prospects: Five-Level Scale for AI Progress
As LLMs continue to evolve, it’s crucial to have a framework for understanding and measuring progress towards more advanced AI capabilities. OpenAI, a leader in AI development, has recently introduced a five-level scale to track progress toward building artificial intelligence software capable of outperforming humans in various tasks.
The Five Levels of AI Capability
Level 1: Conversational AI This level represents the current state of AI, where systems can interact with humans using natural language. LLMs like GPT-4o and Claude 3.5 Sonnet, which we’ve discussed throughout this article, fall into this category.
Level 2: Reasoners The next step in AI evolution involves systems that can perform basic problem-solving tasks as well as a human with a doctorate-level education who doesn’t have access to any tools. OpenAI believes it is on the cusp of reaching this level.
Level 3: Agents At this level, AI systems would be capable of spending several days taking actions on a user’s behalf, demonstrating extended autonomy and task completion abilities.
Level 4: Innovators This advanced level describes AI that can come up with new innovations, pushing beyond human-level problem-solving to create novel solutions and ideas.
Level 5: Organizations The most advanced level in OpenAI’s framework refers to AI systems that can do the work of an entire organization, representing a level of capability and coordination far beyond current AI.
Current Progress and Future Implications
OpenAI executives have indicated that the company believes it is currently at Level 1 but approaching Level 2. This assessment suggests that we may soon see AI systems demonstrating more sophisticated reasoning capabilities, potentially revolutionizing fields like social consumer insights even further. The development of this scale highlights the rapid pace of AI advancement and the industry’s focus on creating increasingly capable systems. As AI progresses through these levels, we can expect to see more profound impacts on various industries, including social consumer insights.
Considerations for the Future
As AI capabilities advance, it’s crucial for businesses and researchers in the field of social consumer insights to consider:
- Ethical Implications: How do we ensure that more advanced AI systems are used responsibly and ethically in analyzing consumer behavior?
- Human-AI Collaboration: How can we best leverage the strengths of both human analysts and AI systems as AI capabilities grow?
- Skill Adaptation: What new skills will professionals in social consumer insights need to develop to work effectively with more advanced AI systems?
- Regulatory Preparedness: How might regulations evolve to address the capabilities of more advanced AI systems in consumer data analysis?
As we look to the future, the continued evolution of AI promises exciting possibilities for social consumer insights. By staying informed about these advancements and thoughtfully considering their implications, businesses can position themselves to leverage these powerful tools effectively while navigating the challenges they may present.
Contact us at [email protected] to discover how our intelligent technologies can propel your business growth.
We look forward to collaborating with you to shape the future!
Relevant Articles:
AI-Powered Consumer Insights
LLMs Transforming Social Listening & Market Insights